ARTIFICIAL INTELLIGENCE

How AI for Physics Connects Technology and Theoretical Physics
What Is AI for Physics?
AI for physics (also “AI physics“) bridges the gap between artificial intelligence and the laws governing the natural world, offering the promise of deeper insights and novel applications across the realm of physics. Both fields underscore the importance of interdisciplinary collaboration and innovation in shaping the future of technology and scientific exploration.
It aims to apply AI techniques to various areas of physics, from analyzing complex physical systems to enhancing our understanding of fundamental laws of the universe.
AI physics leverages machine learning algorithms to simulate, predict, and optimize physical phenomena. Neural networks and other AI models can aid in solving complex differential equations, predicting quantum mechanical behaviors, and even optimizing experimental setups.
This fusion of AI and physics has the potential to accelerate scientific discoveries, streamline data analysis in particle physics and astronomy, and lead to innovations in materials science and energy research. As AI physics evolves, it also raises philosophical questions about the nature of intelligence, the relationship between machine and human understanding of the universe, and the potential insights that machines might uncover in the realm of fundamental physics.
Applications of AI in Physics
In this section, we’ll explore the tangible ways AI is being used in the field of physics. From simulating intricate physical systems to analyzing massive datasets in high-energy physics experiments, AI is accelerating discoveries and pushing the boundaries of our understanding. We’ll also delve into the exciting realm of quantum computing and its intersection with AI, paving the way for quantum advancements that were once thought impossible.
AI has revolutionized the field of physics by introducing innovative applications that enhance our understanding of complex physical phenomena and accelerate the discovery process.
One prominent application is in the realm of particle physics, where AI-driven techniques aid in the analysis of massive datasets generated by particle colliders like the Large Hadron Collider (LHC). Machine learning algorithms excel at identifying elusive particle signatures amidst noisy data, enhancing the accuracy of particle identification and enabling the discovery of rare events that could potentially unveil new fundamental particles or interactions.
Another promising application is computational physics and materials science. AI-driven simulations are transforming the way researchers model and predict the behavior of materials at various scales, from atomic interactions to macroscopic properties. These simulations not only save time but also offer insights into the properties of materials that are challenging to investigate experimentally.
Such AI-powered simulations have contributed to the design of novel materials with tailored properties for applications in electronics, energy storage, and even drug discovery.
Furthermore, AI plays a crucial role in advancing astrophysics by analyzing the vast amount of data collected from telescopes and space missions. Machine learning techniques aid in the identification of celestial objects, the characterization of their properties, and the discovery of new astronomical phenomena.
These algorithms help astronomers sift through enormous datasets, identifying rare events such as gravitational waves or transient cosmic events. AI also contributes to the development of sophisticated models for cosmological simulations, allowing researchers to better understand the evolution of the universe and the distribution of dark matter and dark energy. As AI continues to evolve, its applications in physics promise to unravel deeper mysteries of the universe and revolutionize the way we conduct scientific research.
Physics-Inspired AI Algorithms

Nature has been an incredible source of inspiration for AI algorithms. In this section, we’ll uncover the algorithms that draw inspiration from physics principles. Genetic algorithms, swarm intelligence, and neural architectures modeled after the human brain showcase the remarkable synergy between AI engineering and physics concepts.
Physics-inspired AI algorithms are a captivating intersection of two distinct yet harmonious realms of knowledge, harnessing the principles that govern the physical universe to enhance artificial intelligence techniques. These algorithms draw inspiration from fundamental concepts in physics, such as optimization, entropy, and conservation laws, to develop novel and efficient solutions to complex problems.
By imbuing AI systems with the elegance and predictive power inherent in the laws of physics, researchers aim to create more adaptable, robust, and interpretable algorithms.
One prime example of physics-inspired AI algorithms is the field of quantum machine learning. By leveraging principles from quantum mechanics, these algorithms exploit the inherent parallelism and superposition properties of quantum states to potentially outperform classical machine learning techniques in specific applications.
Quantum-inspired neural networks, for example, can process information in a highly parallel manner, mirroring the behavior of quantum systems, to accelerate tasks like optimization and pattern recognition.
The marriage of quantum physics and AI promises to usher in a new era of computational capabilities, revolutionizing industries such as cryptography and drug discovery, as well as optimization problems that classical computers struggle to solve efficiently.
Physics-inspired AI algorithms also find a home in swarm intelligence and evolutionary computing. Drawing inspiration from the collective behaviors observed in natural systems, these algorithms emulate phenomena like flocking birds, schooling fish, and foraging ants.
By simulating interactions between individual agents and their environment, these algorithms optimize for emergent behaviors, allowing for efficient problem-solving in scenarios where traditional methods might falter. Such approaches hold promise in tasks like optimization, routing and resource allocation, where decentralized decision-making and adaptability are key factors.
Ultimately, the marriage of physics and AI not only enriches our understanding of both domains but also opens up new avenues for solving intricate challenges across a wide spectrum of disciplines.
Bringing Artificial Intelligence (AI) and Physics Together

The intersection of artificial intelligence (AI) and physics lays the foundation for groundbreaking advancements in both fields. At its core, AI draws inspiration from the fundamental principles of physics to model and simulate complex systems, helping machines learn, adapt, and make intelligent decisions.
One of the key connections between AI and physics is the concept of optimization. Just as physical systems tend to evolve towards states of minimum energy or maximum stability, AI algorithms often seek optimal solutions by minimizing or maximizing objective functions. This parallel between the optimization principles in physics and AI has led to the development of algorithms like simulated annealing and genetic algorithms that harness the power of physics-inspired processes to solve complex problems.
Physics offers a wealth of mathematical tools that underpin various AI techniques. Probability theory and statistical methods (essential in both fields) facilitate the modeling of uncertainty and noise. Bayesian networks, for example, find applications in both physics simulations and machine learning tasks such as probabilistic reasoning and decision-making.
Quantum mechanics, a foundational theory in physics, has also started to intersect with AI through the field of quantum machine learning. Quantum computers promise to revolutionize AI by efficiently solving problems that are currently intractable for classical computers, paving the way for advancements in optimization, cryptography, and data analysis.
The bond between AI and physics extends beyond theoretical connections, as physics-driven data plays a pivotal role in training and testing AI models. Physics simulations generate vast datasets that help AI systems learn and adapt in virtual environments.
For example, AI-powered robots learn locomotion skills through simulations that adhere to the laws of physics, helping them transfer their knowledge to the real world. Similarly, in fields like astrophysics and materials science, AI aids researchers in sifting through immense data volumes to identify patterns, anomalies, and new discoveries that would be otherwise challenging to discern manually.
AI’s Role in Solving Grand Physics Challenges
AI has emerged as a transformative tool in tackling some of the most perplexing grand challenges in physics. With its capacity to process vast amounts of data and generate intricate models, AI has helped physicists unravel complex phenomena that were previously beyond human comprehension.
In particle physics, AI-driven algorithms have played a pivotal role in analyzing high-energy collisions and identifying elusive subatomic particles, advancing our understanding of the fundamental building blocks of the universe. Moreover, AI has expedited progress in cosmology by simulating intricate cosmological models and aiding in the interpretation of astronomical observations. Additionally, in the realm of quantum mechanics, AI has contributed to optimizing quantum computing algorithms and deciphering intricate quantum states.
As AI continues to evolve, it holds the promise of further breakthroughs, accelerating our comprehension of the intricate workings of the cosmos and propelling us toward solving some of physics’ most enigmatic puzzles.
Ethical Considerations of AI in Physics
AI is making significant contributions to the field of physics, aiding in data analysis, simulations, and discovery. However, this integration raises ethical questions that must be addressed.
One concern is the appropriate use of AI-generated results and discoveries. While AI can help researchers uncover patterns and correlations in complex data sets, there’s a need to ensure that these findings are validated through traditional scientific methods.
The risk of overreliance on AI-generated insights without proper verification could lead to erroneous conclusions. Moreover, ethical challenges arise in cases where AI is employed to predict the outcomes of physics experiments or simulations. The question of whether AI predictions should be treated as valid hypotheses or mere predictions requires careful consideration.
The Future of AI Physics
AI’s role in tackling grand challenges in physics, such as understanding dark matter and cosmic inflation, will shape the next generation of scientific breakthroughs.
The future unfolds with a deepening understanding of the fundamental principles governing artificial intelligence systems. AI physics seeks to elucidate the underlying mathematical and physical principles that guide the behavior of neural networks, optimization algorithms, and learning processes.
This emerging field will provide insights into the generalization capabilities of AI models, helping researchers design architectures that are capable of more demanding tasks while being reliable and accurate.
As AI systems become more complex and interconnected, the application of AI physics will be pivotal in ensuring their stability, security, and ethical use, fostering a deeper grasp of how these systems interact with their environment and adapt to new challenges.
Advancement of Quantum AI
In the rapidly evolving landscape of quantum AI, recent years have witnessed remarkable breakthroughs that are reshaping the boundaries of computational capabilities. Researchers and engineers have made significant strides in harnessing the unique properties of quantum systems to enhance artificial intelligence processes.
Quantum neural networks, which exploit quantum entanglement and superposition, now demonstrate unparalleled performance in tasks like optimization, pattern recognition, and complex data analysis. The development of more stable and error-tolerant quantum processors has paved the way for the realization of large-scale quantum AI systems, enabling the exploration of intricate problem spaces that were previously intractable.
As quantum AI continues to mature, its transformative potential spans diverse domains, from drug discovery and materials science to cryptography and optimization, propelling us into an era where the synergy between quantum mechanics and artificial intelligence reshapes the technological landscape.
Emergence of Quantum Machine Learning
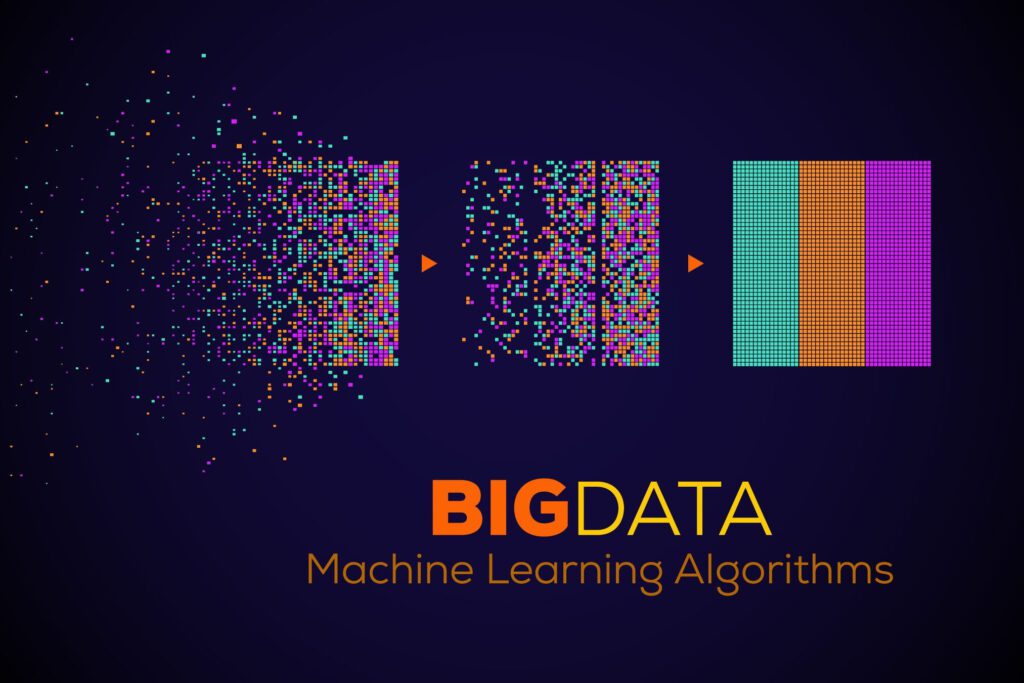
The emergence of quantum machine learning (QML) stands as a revolutionary convergence of two cutting-edge fields: quantum computing and machine learning. QML harnesses the unique properties of quantum systems, such as superposition and entanglement, to redefine the boundaries of computational capabilities. By leveraging these quantum phenomena, QML algorithms hold the promise of solving complex optimization, pattern recognition, and data analysis problems exponentially faster than classical counterparts.
This novel paradigm opens doors to accelerating the training of deep neural networks, enhancing drug discovery, optimizing financial portfolios, and tackling intricate optimization challenges. As quantum hardware and algorithms advance, the synergy between quantum computing and machine learning is poised to reshape industries and scientific frontiers, paving the way for transformative advancements in the realm of artificial intelligence.
Relativity
Relativity, a cornerstone of modern physics, has intriguing implications for the foundations of AI. Albert Einstein’s theory of relativity, comprising both special and general relativity, fundamentally altered our understanding of space, time, and gravity.
In the context of AI, relativity’s significance lies in its emphasis on the interconnectedness of various perspectives and frames of reference. Similarly, in the realm of artificial intelligence, the concept of relativity underscores the importance of diverse data sources, viewpoints, and contextual understanding.
Just as Einstein’s theory shattered classical notions of absolute space and time, AI systems are challenged to dynamically adapt and learn from changing environments, embracing relativistic adaptability. Incorporating relativity-inspired principles into AI could lead to more robust, flexible, and contextually aware systems capable of navigating complex and ever-evolving scenarios with enhanced cognitive agility.
Thermodynamics

Thermodynamics, a fundamental branch of physics that explores the behavior of energy and heat, may seem distant from the realms of artificial intelligence (AI) at first glance. However, the parallels between the two fields become evident when delving deeper.
Just as thermodynamics governs the flow and transformation of energy within physical systems, the principles of AI rest upon the manipulation and conversion of information. The concept of entropy, central to thermodynamics, finds an intriguing counterpart in the context of AI—reflecting the tendency of systems to evolve towards disorder.
Similarly, the efficient utilization of resources and the optimization of processes, crucial in both fields, draw inspiration from the efficiency goals of thermodynamic cycles. In understanding the foundations of AI, an appreciation of thermodynamics offers insights into the intricate interplay between information, computation, and the underlying principles that shape our ever-evolving technological landscape.
CREDITS: https://rescale.com/blog/ai-physics/
PINN’s Physics Informed Neural Network
Physics-informed neural networks (PINNs) are a type of universal function approximators that can embed the knowledge of any physical laws that govern a given data-set in the learning process, and can be described by partial differential equations (PDEs). They overcome the low data availability of some biological and engineering systems that makes most state-of-the-art machine learning techniques lack robustness, rendering them ineffective in these scenarios.[1] The prior knowledge of general physical laws acts in the training of neural networks (NNs) as a regularization agent that limits the space of admissible solutions, increasing the correctness of the function approximation. This way, embedding this prior information into a neural network results in enhancing the information content of the available data, facilitating the learning algorithm to capture the right solution and to generalize well even with a low amount of training examples.

ROBOTICS

Robotics in Healthcare: The Future of Robots in Medicine
Intelligent Automation Technology for Healthcare
Emerging in the 1980s, the first robots in the medical field provided surgical assistance via robotic arm technologies. Over the years, artificial intelligence (AI)–enabled computer vision and data analytics have transformed medical robots, expanding their capabilities into many other areas of healthcare.
Robots are now used not only in the operating room but also in clinical settings to support healthcare workers and enhance patient care. For example, hospitals and clinics are deploying robots for a much wider range of tasks to help reduce exposure to pathogens during the COVID-19 pandemic.
The use of robotics and automation also extends to research laboratories where they are used to automate manual, repetitive, and high-volume tasks so technicians and scientists can focus their attention on more strategic tasks that make discoveries happen faster.
Streamlined workflows and risk reduction provided by medical robotics offer value in many areas. For example, robots can clean and prep patient rooms independently, helping limit person-to-person contact in infectious disease wards. Robots with AI-enabled medicine identifier software reduce the time it takes to identify, match, and distribute medicine to patients in hospitals.
As technologies evolve, robots will function more autonomously, eventually performing certain tasks entirely on their own. As a result, doctors, nurses, and other healthcare workers will be able to spend more time providing direct patient care.
Benefits of Robotics in Healthcare
Using robotics in the medical field enables a high level of patient care, efficient processes in clinical settings, and a safe environment for patients and healthcare workers.
High-Quality Patient Care
Medical robots support minimally invasive procedures, customized and frequent monitoring for patients with chronic diseases, intelligent therapeutics, and social engagement for elderly patients. In addition, as robots alleviate workloads, nurses and other caregivers can offer patients more empathy and human interaction, which can promote long-term well-being.
Streamlined Clinical Workflows
Autonomous mobile robots (AMRs) simplify routine tasks, reduce the physical demands on human workers, and ensure more consistent processes. These robots can address staffing shortages and challenges by keeping track of inventory and placing timely orders to help make sure supplies, equipment, and medication are in stock where they are needed. Cleaning and disinfection AMRs enable hospital rooms to be sanitized and ready for incoming patients quickly, allowing workers to focus on patient-centric, value-driven work.
Safe Work Environment
To help keep healthcare workers safe, AMRs are used to transport supplies and linens in hospitals where pathogen exposure is a risk. Cleaning and disinfection robots limit pathogen exposure while helping reduce hospital acquired infections (HAIs)—and hundreds of healthcare facilities are already using them1. Social robots, a type of AMR, also help with heavy lifting, such as moving beds or patients, which reduces physical strain on healthcare workers.
Surgical-Assistance Robots
As motion control technologies have advanced, surgical-assistance robots have become more precise. These robots help surgeons achieve new levels of speed and accuracy while performing complex operations with AI- and computer vision‒capable technologies. Some surgical robots may even be able to complete tasks autonomously, allowing surgeons to oversee procedures from a console.
Surgeries performed with robotics assistance fall into two main categories:
- Minimally invasive surgeries for the torso. These include robotic hysterectomy, robotic prostatectomy, bariatric surgery, and other procedures primarily focused on soft tissues. After insertion through a small incision, these robots lock themselves into place, creating a stable platform from which to perform surgeries via remote control. Open surgery using large incisions was once the norm for most internal procedures. Recovery times were much longer, and the potential for infection and other complications was greater. Working manually through a button-sized incision is extremely difficult, even for an experienced surgeon. Surgical robots make these procedures easy and accurate, with a goal to reduce infections and other complications.
- Orthopedic surgeries. Devices can be preprogrammed to perform common orthopedic surgeries, such as knee and hip replacements. Combining smart robotic arms, 3D imaging, and data analytics, these robots enable more predictable results by employing spatially defined boundaries to assist the surgeon. AI modeling enables robots to be trained in specific orthopedic surgeries, with precise direction for where to go and how to perform the procedures.
The ability to share a video feed from the operating room to other locations—near or far—allows surgeons to benefit from consultations with other specialists in their field. As a result, patients have the best surgeons involved in their procedures.
The field of surgical robotics is evolving to make greater use of AI. Computer vision enables surgical robots to differentiate between types of tissue within their field of view. For example, surgical robots now have the ability to help surgeons avoid nerves and muscles during procedures2. High-definition 3D computer vision can provide surgeons with detailed information and enhanced performance during procedures. Eventually, robots will be able to take over small subprocedures, such as suturing or other defined tasks, under the watchful gaze of the surgeon.
Robotics also plays a key role in surgeon education. Simulation platforms use AI and virtual reality to provide surgical robotics training. Within the virtual environment, surgeons can practice procedures and hone skills using robotics controls.
Modular Robots
Modular robots enhance other systems and can be configured to perform multiple functions. In healthcare, these include therapeutic exoskeleton robots and prosthetic robotic arms and legs.
Therapeutic robots can help with rehabilitation after strokes, paralysis, or traumatic brain injuries or with impairments caused by multiple sclerosis. A wheelchair-mounted robotic arm currently being developed by Intel and Accenture aims to assist patients with spinal injuries in performing daily tasks. When robots are equipped with AI and depth cameras, they can monitor a patient’s form as they go through prescribed exercises, measuring degrees of motion in different positions and tracking progress more precisely than the human eye. They can also interact with patients to provide coaching and encouragement.
Autonomous Mobile Robots
Healthcare organizations often rely on AMRs because of their ability to assist with critical needs such as disinfection, telepresence, and delivery of medication and medical supplies, creating safe environments while freeing up staff to spend more time with patients. When equipped with light detection and ranging (LiDAR) systems, visual compute, or mapping capabilities, AMRs can self-navigate to patients in exam or hospital rooms, allowing clinicians to interact from afar. If an AMR is controlled by a remote specialist or other worker, it can accompany doctors as they make hospital rounds, allowing a specialist to contribute via an on-screen consultation regarding patient diagnostics and care.
Some robots can assist professionals before a patient is checked in. For example, one autonomous robot in Mexico, developed by start-up Roomie, is being used to help medical staff with high-risk COVID-19 patients. Named RoomieBot, it triages patients by taking their temperature, blood oxygen level, and medical history when they arrive at the hospital. RoomieBot uses Intel-based technology, including AI algorithms that run on the Intel® Movidius™ Vision Processing Unit (VPU), 8th Gen Intel® NUCs, and Intel® RealSense™ cameras.
Other types of AMRs that are used in healthcare include service robots and social robots.
Service Robots
Service robots relieve the daily burden on healthcare workers by handling routine logistical tasks. Many of these robots function autonomously and can send a report when they complete a task. These robots set up patient rooms, track supplies and file purchase orders, restock medical supply cabinets, and transport bed linens to and from laundry facilities. Having some routine tasks performed by service robots gives healthcare workers more time to focus on immediate patient needs and can help with increasing job satisfaction. The TUG Robot by Aethon is a service robot doing just that. TUG can navigate complex and changing environments to safely deliver linens to nursing units on both a scheduled and on-demand basis.
Service robots can also help with cleaning and disinfection. These AMRs may use ultraviolet (UV) light, hydrogen peroxide vapors, or air filtration to help reduce infection and to sanitize reachable places in a uniform way. An autonomous mobile robot prototype developed by the start-up Akara is being tested for one of these routine yet essential tasks: disinfecting contaminated surfaces using UV light. Its goal is to help hospitals sanitize rooms and equipment, aiding in the fight against COVID-19.
Social Robots
Social robots interact directly with humans. These “friendly” robots can be used in long-term care environments to provide social interaction and monitoring. They may encourage patients to comply with treatment regimens or provide cognitive engagement, helping to keep patients alert and positive. They can also be used to offer directions to visitors and patients inside the hospital environment. In general, social robots help reduce caregiver workloads and improve patients’ emotional well-being.